e all tend to follow habits at work and in other aspects of our lives. I generally work best in the morning and hate working after dinner. Our habits usually reflect preferences, learning, or a combination of the two – or, as Charles Duhigg notes in his book The Power of Habit, the mere repetition of an act might generate a routine.
My own recent research shows that criminals are not all that different from law-abiding citizens when it comes to sticking to a routine, possibly as a result of their experience, their tendency to specialize, and their belief that they have developed an ideal strategy. And police departments are catching on, with the assistance of an increasingly common tool: algorithms.
Because algorithms use patterns in data to predict future behavior, they can forecast the movies someone might like on Netflix or the books she might buy on Amazon. But they can also help law-enforcement agencies fight crime. Some algorithms calculate prison inmates’ future recidivism. Others underpin predictive-policing tools, which generate crime forecasts with the aim of optimizing patrols.
Investments in predictive analytics lead to a reallocation of resources (including through different policing strategies and alternative sentencing), and thus alter the probability of arrest or detention across individuals. For this reason, it is important to understand whether these algorithmic tools reduce crime, and whether they do so without generating biases against certain groups.
The most elaborate and well-known predictive-policing software has essentially evolved from hotspot maps. These programs operate on the principle that areas recently subject to high crime are more likely to have high near-term crime rates. Thus, law-enforcement agencies should focus on these areas in order to deter the largest number of criminals.
While researchers have shown that these statistical algorithms have greater predictive power than simple averages, proving that they actually reduce crime is considerably more difficult. Police departments tend to embrace predictive policing when crime is high, and subsequent reductions might reflect a natural decline that has nothing to do with that decision. Area-focused policing may also simply shift crime elsewhere. A proper evaluation therefore requires a better counterfactual scenario: What would have happened to crime without the use of predictive policing?
As for bias, it is not inconceivable that predictive policing may distort law-enforcement outcomes. More deprived areas may have higher crime rates and will be patrolled more intensively once predictive policing is introduced. If police resources remain fixed, criminals in deprived areas will have a greater chance of encountering a police patrol than criminals in more affluent neighborhoods. But while this is a fair outcome for serial offenders who contributed to the spike in crime that led to the extra patrolling, today’s first-time offenders did not previously increase the crime numbers. Because most predictive-policing algorithms bundle together crime incidents without separating habitual criminals from first-time offenders, they may be biased against the latter in deprived areas.
To help address the questions of effectiveness and bias, I evaluated predictive-policing software used in Milan, Italy. This allowed me to establish a proper counterfactual: For historical reasons, Milan has two police departments that share the same objectives, but only one of them uses predictive policing.
KeyCrime, the predictive software developed by Mario Venturi that is used in Milan, differs from common predictive-policing tools because it focuses on arresting perpetrators rather than deterring them (thus denying criminals the opportunity simply to go somewhere else) and distinguishes first-time offenders from recurrent criminals. The software uses information gathered from victim reports and CCTV cameras to link criminals to commercial robberies, and then predicts when and where a particular individual or group will strike next. KeyCrime generates individual predictions, which reduces the scope for bias.
The results indicate that analyzing the habits of recurrent criminals more than doubles the likelihood of arresting them. Thieves tend to act in a similar way over time, targeting a specific neighborhood and type of business, as well as sticking to a certain time of day. So, for example, someone who previously robbed a jewelry shop at nine o’clock in the morning is likely to reoffend in the same neighborhood, at around the same time, and against another jeweler. Since there are only so many matches that fit the predictions, the software highlights the potential future targets, and the police department organizes patrols to catch the thief.
Micro-predictions based on individual criminal groups’ behavior have been proven to work in combating robberies and are now being extended to other types of serial offenders, like sex offenders and terrorists. Whether predictive policing will be equally successful in bringing these criminals to justice remains to be seen, as reduced interaction with victims and availability of CCTV footage might make it more difficult to link offenders across incidents.
More widespread use of predictive policing may lead criminals to change their habits and become less predictable. But the development of more powerful algorithms and data-gathering processes provides some grounds for optimism among police departments.
“Crime is terribly revealing,” Agatha Christie wrote. “Try and vary your methods as you will, your tastes, your habits, your attitude of mind, and your soul is revealed by your actions.” For now, building capacity to predict individual crimes by using criminals’ habits against them appears to be a good investment.
Copyright: Project Syndicate, 2022.
a global affairs media network
Do Algorithms Help to Reduce Crime?
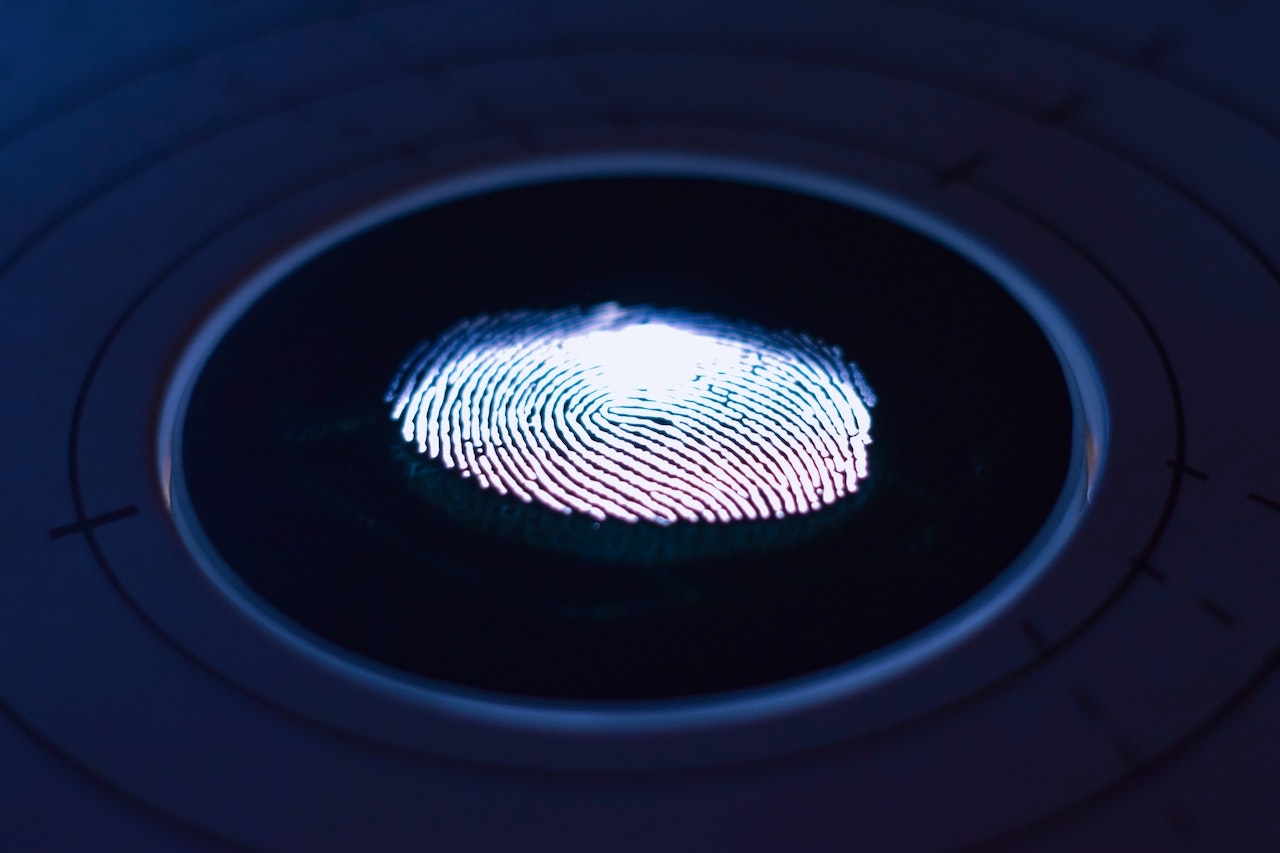
Photo by George Prentzas via Unsplash.
May 23, 2022
Criminals are not all that different from law-abiding citizens when it comes to sticking to a routine. Police departments are catching on to this with the assistance of an increasingly common tool: algorithms, writes Professor of Economics at the University of Essex Giovanni Mastrobuoni.
W
e all tend to follow habits at work and in other aspects of our lives. I generally work best in the morning and hate working after dinner. Our habits usually reflect preferences, learning, or a combination of the two – or, as Charles Duhigg notes in his book The Power of Habit, the mere repetition of an act might generate a routine.
My own recent research shows that criminals are not all that different from law-abiding citizens when it comes to sticking to a routine, possibly as a result of their experience, their tendency to specialize, and their belief that they have developed an ideal strategy. And police departments are catching on, with the assistance of an increasingly common tool: algorithms.
Because algorithms use patterns in data to predict future behavior, they can forecast the movies someone might like on Netflix or the books she might buy on Amazon. But they can also help law-enforcement agencies fight crime. Some algorithms calculate prison inmates’ future recidivism. Others underpin predictive-policing tools, which generate crime forecasts with the aim of optimizing patrols.
Investments in predictive analytics lead to a reallocation of resources (including through different policing strategies and alternative sentencing), and thus alter the probability of arrest or detention across individuals. For this reason, it is important to understand whether these algorithmic tools reduce crime, and whether they do so without generating biases against certain groups.
The most elaborate and well-known predictive-policing software has essentially evolved from hotspot maps. These programs operate on the principle that areas recently subject to high crime are more likely to have high near-term crime rates. Thus, law-enforcement agencies should focus on these areas in order to deter the largest number of criminals.
While researchers have shown that these statistical algorithms have greater predictive power than simple averages, proving that they actually reduce crime is considerably more difficult. Police departments tend to embrace predictive policing when crime is high, and subsequent reductions might reflect a natural decline that has nothing to do with that decision. Area-focused policing may also simply shift crime elsewhere. A proper evaluation therefore requires a better counterfactual scenario: What would have happened to crime without the use of predictive policing?
As for bias, it is not inconceivable that predictive policing may distort law-enforcement outcomes. More deprived areas may have higher crime rates and will be patrolled more intensively once predictive policing is introduced. If police resources remain fixed, criminals in deprived areas will have a greater chance of encountering a police patrol than criminals in more affluent neighborhoods. But while this is a fair outcome for serial offenders who contributed to the spike in crime that led to the extra patrolling, today’s first-time offenders did not previously increase the crime numbers. Because most predictive-policing algorithms bundle together crime incidents without separating habitual criminals from first-time offenders, they may be biased against the latter in deprived areas.
To help address the questions of effectiveness and bias, I evaluated predictive-policing software used in Milan, Italy. This allowed me to establish a proper counterfactual: For historical reasons, Milan has two police departments that share the same objectives, but only one of them uses predictive policing.
KeyCrime, the predictive software developed by Mario Venturi that is used in Milan, differs from common predictive-policing tools because it focuses on arresting perpetrators rather than deterring them (thus denying criminals the opportunity simply to go somewhere else) and distinguishes first-time offenders from recurrent criminals. The software uses information gathered from victim reports and CCTV cameras to link criminals to commercial robberies, and then predicts when and where a particular individual or group will strike next. KeyCrime generates individual predictions, which reduces the scope for bias.
The results indicate that analyzing the habits of recurrent criminals more than doubles the likelihood of arresting them. Thieves tend to act in a similar way over time, targeting a specific neighborhood and type of business, as well as sticking to a certain time of day. So, for example, someone who previously robbed a jewelry shop at nine o’clock in the morning is likely to reoffend in the same neighborhood, at around the same time, and against another jeweler. Since there are only so many matches that fit the predictions, the software highlights the potential future targets, and the police department organizes patrols to catch the thief.
Micro-predictions based on individual criminal groups’ behavior have been proven to work in combating robberies and are now being extended to other types of serial offenders, like sex offenders and terrorists. Whether predictive policing will be equally successful in bringing these criminals to justice remains to be seen, as reduced interaction with victims and availability of CCTV footage might make it more difficult to link offenders across incidents.
More widespread use of predictive policing may lead criminals to change their habits and become less predictable. But the development of more powerful algorithms and data-gathering processes provides some grounds for optimism among police departments.
“Crime is terribly revealing,” Agatha Christie wrote. “Try and vary your methods as you will, your tastes, your habits, your attitude of mind, and your soul is revealed by your actions.” For now, building capacity to predict individual crimes by using criminals’ habits against them appears to be a good investment.
Copyright: Project Syndicate, 2022.