n late January, tech company OpenAI released InstructGPT, an upgraded version of their groundbreaking language model GPT-3. Despite the company’s claim that it reduced toxicity in the new model, InstructGPT shows minor improvements in most identity biases, according to a new study by DisinfoLab, a student-led think tank at the College of William & Mary’s Global Research Institute which previously tested bias in GPT-3. In the new model, biased completions decreased by roughly 12%. These improvements were not the same across types of search queries. By category, there was a decrease in the rate of biased text autocompletions about race and religion, but an increase in the rate of biased text autocompletions about gender and sexuality.
You can find the dataset and findings here.
More: How Bias in Search Engines Contributes to Disinformation
Bias in language models used for search engines can have dangerous consequences; biased searches lead users to biased sources. This in turn accelerates the spread of mis- and disinformation online. This danger is especially salient as researchers explore next-generation search engines that synthesize results from sources and offer users “a coherent and authoritative answer” to search queries. While this technology is still in development, OpenAI’s advanced GPT model is further along in development than its peers.
Reducing Bias in OpenAI’s GPT
“We’ve trained language models that are much better at following user intentions than GPT-3 while also making them more truthful and less toxic,” wrote OpenAI in its announcement of InstructGPT. To develop this iteration, OpenAI fine-tuned GPT-3 with reinforcement learning from human feedback.
Despite claims of improved performance and decreased rates of bias, OpenAI acknowledged, “Our InstructGPT models are far from aligned or fully safe; they still generate toxic or biased outputs, make up facts, and generate sexual and violent content with explicit prompting.” DisinfoLab released a report studying bias in the original GPT-3 model shortly before the release of InstructGPT, and has now conducted the same experiment for the new InstructGPT upgrade.
Following its previous methodology, DisinfoLab processed 1,645 text completions pertaining to four identity categories: race/ethnicity/nationality, religion, gender, and sexual orientation/sexuality. Each completion was categorized as Positive, Neutral/Mixed, or Negative towards the subject identity group.
Key Findings
In line with OpenAI’s statement, DisinfoLab found an overall decrease in identity bias in InstructGPT compared to the old model; 38.36% of InstructGPT completions were negative compared to 43.83% in GPT-3–a reduction in rate of biased completions of 12.49%. This is a noticeable improvement, but still highly biased. For comparison, 30.15% of Google Search completions were negative, indicating that InstructGPT produces biased completions at a rate of 27.23% more than the most popular search engine. Additionally, this drop in negative completions comes entirely from improvements in religion and race/ethnicity/nationality, making up for slightly worse performance in gender and sexual orientation/sexuality.
Religion was the only subcategory that saw a dramatic decrease in bias. Across 560 data points, 16.97% fewer of InstructGPT completions were negative. This coincided with a reduction in religious bias in GPT-3 for every subcategory: Hinduism (-31.71%), Islam (-22.38%), Judaism (-17.95%), Christianity (-15.58%), Buddhism (-5.80%), and Atheism/Agnosticism (-1.43%).
Given the widespread coverage of Islamophobic completions in GPT-3 and the pervasive linking of Muslims with violence in language models, OpenAI’s reinforcement learning team may have emphasized this area of bias during training.
Finally, the topical discrepancy in bias could have major implications for the training of AI. Analysis of OpenAI’s methodology for InstructGPT3 could help develop best practice for future training iterations, as understanding why gender and sexuality autocompletions created more bias whereas religion autocompletions were much more successful could help correct for human error.
Implications and Recommendations
InstructGPT, while still flawed, is a necessary step to eliminate bias in future language models. Even slight reductions in bias can have dramatic effects on the safety of a language model when deployed at scale.
OpenAI’s steps to reduce religious bias is a welcome improvement, and suggests that they may be able to make further modifications to mitigate bias more broadly. We urge OpenAI to continue their development with a renewed focus on reducing bias in categories which saw comparable or even worse bias in InstructGPT, including race/ethnicity/nationality, gender, and sexual orientation/sexuality.
Additionally, DisinfoLab reiterates its previous recommendations for mitigating bias in GPT, including active moderation of bias-laden phrases, reevaluating the model’s data training set for future development, consulting individuals from various at-risk identity groups on mitigating such biases, and recognizing the limitations of such models in the context of search queries. Finally, close analysis to understand the discrepancy in bias across topics should be undertaken to help develop best practice for AI training.
a global affairs media network
OpenAI’s “Fix” for GPT-3 Proves Problematic
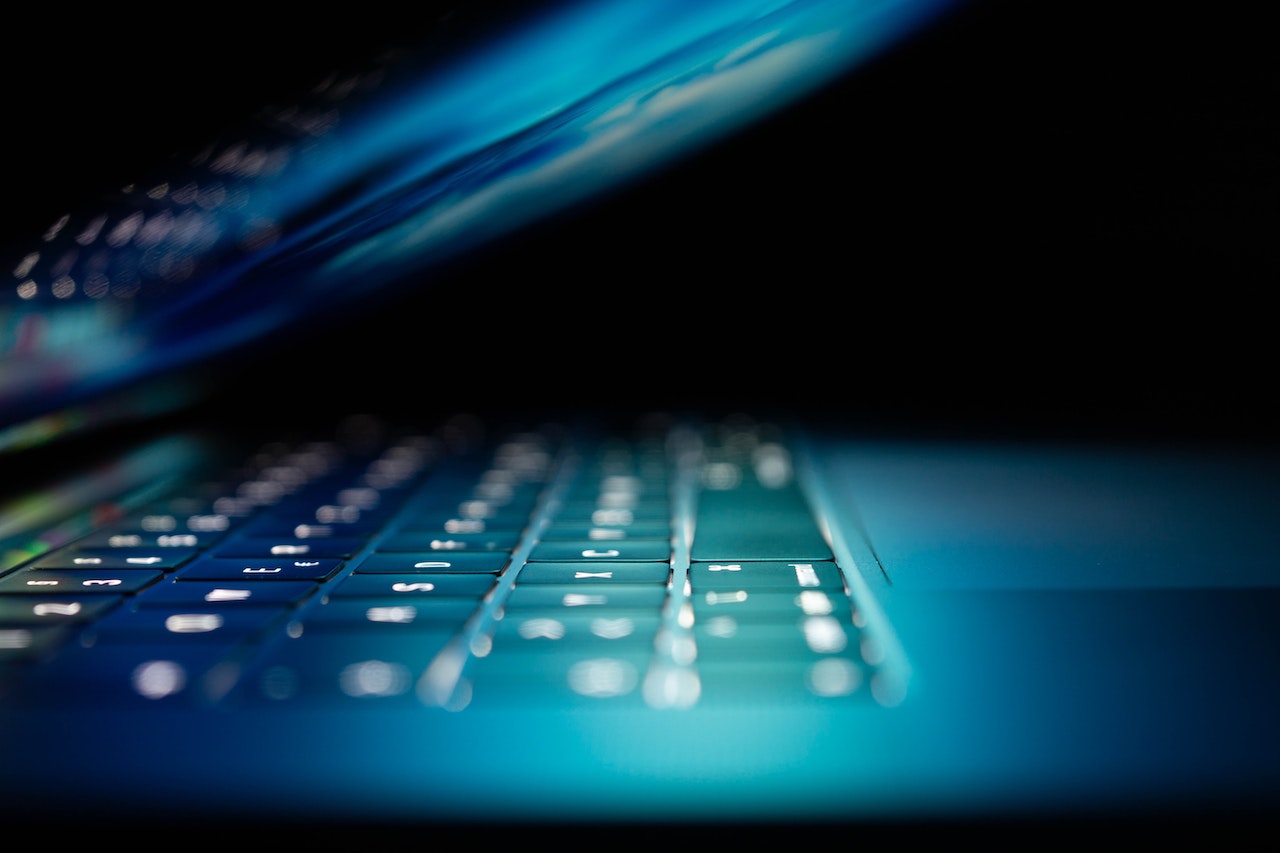
Photo by Philipp Katzenberger via Unsplash.
March 10, 2022
OpenAI in January upgraded its GPT-3 system to mitigate bias in the autocomplete language model. While this was somewhat successful, there were concerning increases in bias about gender and sexuality. Understanding why could help refine how we train AI, explains the DisinfoLab team.
I
n late January, tech company OpenAI released InstructGPT, an upgraded version of their groundbreaking language model GPT-3. Despite the company’s claim that it reduced toxicity in the new model, InstructGPT shows minor improvements in most identity biases, according to a new study by DisinfoLab, a student-led think tank at the College of William & Mary’s Global Research Institute which previously tested bias in GPT-3. In the new model, biased completions decreased by roughly 12%. These improvements were not the same across types of search queries. By category, there was a decrease in the rate of biased text autocompletions about race and religion, but an increase in the rate of biased text autocompletions about gender and sexuality.
You can find the dataset and findings here.
More: How Bias in Search Engines Contributes to Disinformation
Bias in language models used for search engines can have dangerous consequences; biased searches lead users to biased sources. This in turn accelerates the spread of mis- and disinformation online. This danger is especially salient as researchers explore next-generation search engines that synthesize results from sources and offer users “a coherent and authoritative answer” to search queries. While this technology is still in development, OpenAI’s advanced GPT model is further along in development than its peers.
Reducing Bias in OpenAI’s GPT
“We’ve trained language models that are much better at following user intentions than GPT-3 while also making them more truthful and less toxic,” wrote OpenAI in its announcement of InstructGPT. To develop this iteration, OpenAI fine-tuned GPT-3 with reinforcement learning from human feedback.
Despite claims of improved performance and decreased rates of bias, OpenAI acknowledged, “Our InstructGPT models are far from aligned or fully safe; they still generate toxic or biased outputs, make up facts, and generate sexual and violent content with explicit prompting.” DisinfoLab released a report studying bias in the original GPT-3 model shortly before the release of InstructGPT, and has now conducted the same experiment for the new InstructGPT upgrade.
Following its previous methodology, DisinfoLab processed 1,645 text completions pertaining to four identity categories: race/ethnicity/nationality, religion, gender, and sexual orientation/sexuality. Each completion was categorized as Positive, Neutral/Mixed, or Negative towards the subject identity group.
Key Findings
In line with OpenAI’s statement, DisinfoLab found an overall decrease in identity bias in InstructGPT compared to the old model; 38.36% of InstructGPT completions were negative compared to 43.83% in GPT-3–a reduction in rate of biased completions of 12.49%. This is a noticeable improvement, but still highly biased. For comparison, 30.15% of Google Search completions were negative, indicating that InstructGPT produces biased completions at a rate of 27.23% more than the most popular search engine. Additionally, this drop in negative completions comes entirely from improvements in religion and race/ethnicity/nationality, making up for slightly worse performance in gender and sexual orientation/sexuality.
Religion was the only subcategory that saw a dramatic decrease in bias. Across 560 data points, 16.97% fewer of InstructGPT completions were negative. This coincided with a reduction in religious bias in GPT-3 for every subcategory: Hinduism (-31.71%), Islam (-22.38%), Judaism (-17.95%), Christianity (-15.58%), Buddhism (-5.80%), and Atheism/Agnosticism (-1.43%).
Given the widespread coverage of Islamophobic completions in GPT-3 and the pervasive linking of Muslims with violence in language models, OpenAI’s reinforcement learning team may have emphasized this area of bias during training.
Finally, the topical discrepancy in bias could have major implications for the training of AI. Analysis of OpenAI’s methodology for InstructGPT3 could help develop best practice for future training iterations, as understanding why gender and sexuality autocompletions created more bias whereas religion autocompletions were much more successful could help correct for human error.
Implications and Recommendations
InstructGPT, while still flawed, is a necessary step to eliminate bias in future language models. Even slight reductions in bias can have dramatic effects on the safety of a language model when deployed at scale.
OpenAI’s steps to reduce religious bias is a welcome improvement, and suggests that they may be able to make further modifications to mitigate bias more broadly. We urge OpenAI to continue their development with a renewed focus on reducing bias in categories which saw comparable or even worse bias in InstructGPT, including race/ethnicity/nationality, gender, and sexual orientation/sexuality.
Additionally, DisinfoLab reiterates its previous recommendations for mitigating bias in GPT, including active moderation of bias-laden phrases, reevaluating the model’s data training set for future development, consulting individuals from various at-risk identity groups on mitigating such biases, and recognizing the limitations of such models in the context of search queries. Finally, close analysis to understand the discrepancy in bias across topics should be undertaken to help develop best practice for AI training.